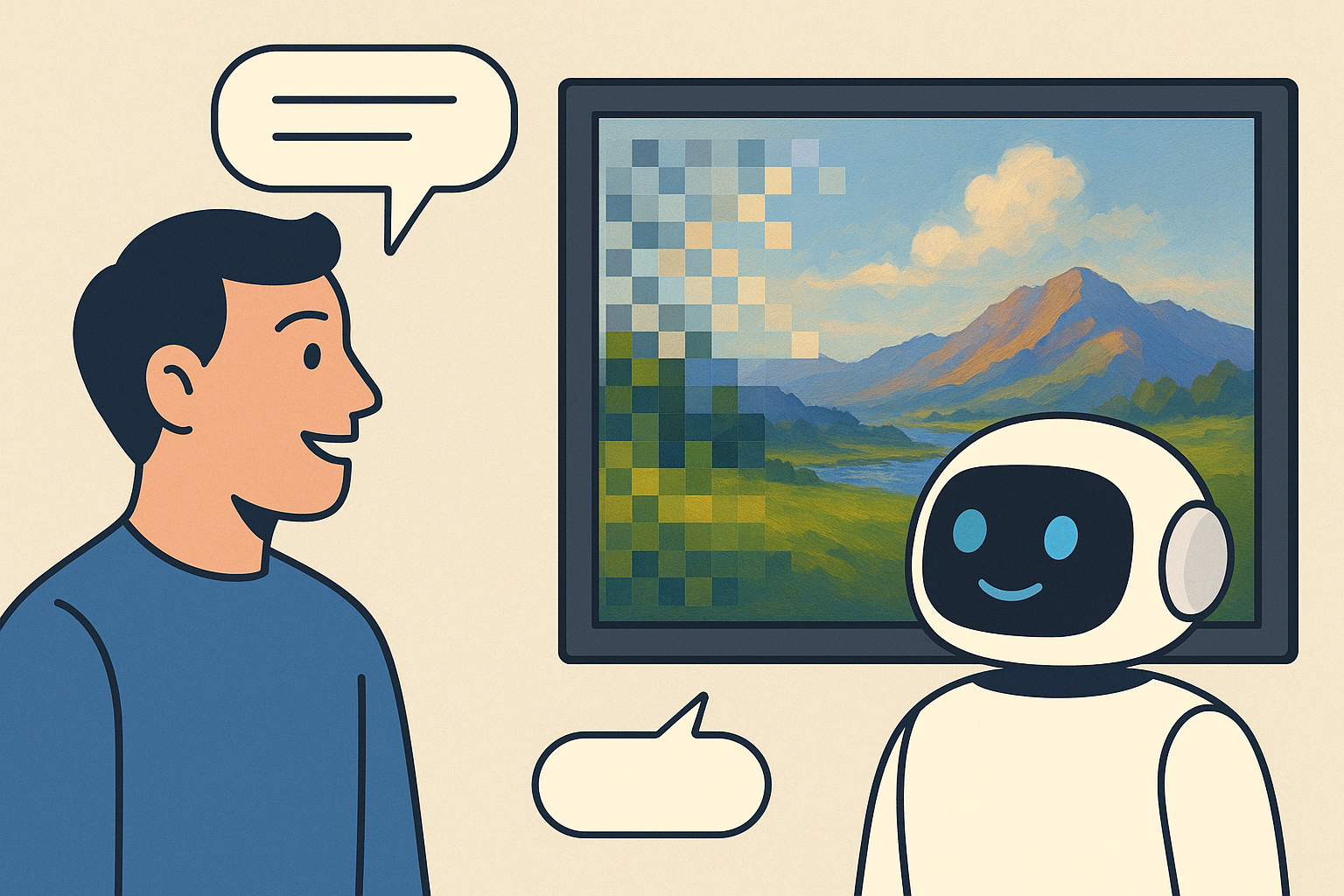
From Prompts to Conversations: A New Creative Workflow
One of the most profound shifts with the rise of autoregressive image models is how we interact with these tools. With classic diffusion tools, you typically write a single prompt – which could be a long string of keywords like “sunset over a tranquil lake, photorealistic, 4K, golden lighting, reflection, –ar 16:9” – and then hit generate. If the result isn’t what you want, you adjust the prompt or some settings and try again, essentially engaging in a guess-and-check process. It required learning the AI’s “language” to get good results consistently, and communities formed around sharing prompt tips.
Now compare that to a conversation-driven approach: you can start with a simple prompt and then refine the image through dialogue. For example, you might tell ChatGPT’s image feature, “a forest at sunset”. The model produces a lovely wooded sunset scene. You then say, “Now add a deer by a stream in the foreground.” The AI understands this as an instruction to modify or regenerate the image with those new elements, and it does so. This iterative loop can continue, allowing you to fine-tune details – “make the deer larger and facing the viewer” – or try stylistic changes – “can you make it look more like an oil painting?”. The ability to talk to your image generator and have it respond in context is a game-changer for creative workflows. It feels less like programming an algorithm and more like collaborating with a smart assistant who just happens to have a vivid imagination and superb art skills.
For artists and designers, this means AI can slot into the creative process more naturally. Instead of spending time wrangling prompts or generating dozens of separate images hoping one is close to your vision, you can steer a single generation in the right direction step by step. It’s akin to giving an art director continuous feedback: “make that color warmer, put a little more detail here, try a different angle.” This also lowers the entry barrier for newcomers. Someone who isn’t versed in prompt engineering or doesn’t know terms like “cinematic lighting” can still get the image they want by simply describing it in plain English and refining interactively. In other words, user experience has evolved from coding prompts to holding conversations.
Another aspect of this new workflow is integration. Because a model like GPT-4o is essentially a unified multimodal AI, you can mix image generation with other tasks. For instance, you could ask for an image and also ask the AI to write a backstory or a description for it in the same session. Or in a single conversation, design a character (with images) and write dialogues for that character. The image and text parts share context with each other. This opens up the possibility of richer creative sessions – imagine brainstorming a children’s story where the AI both writes the narrative and generates illustrations on the fly, with you guiding both aspects. We’re already seeing early glimpses of such workflows. Developers are building plugins for chat platforms where the AI can switch between showing text and images in its response. The creative process becomes fluid: you don’t have to leave the chat to use a separate art tool, it’s all intertwined.
This shift also affects collaboration. In a team setting, not everyone needs to be adept at using an image generator tool separately. Instead, during a meeting, team members could collectively chat with an AI on a big screen to hash out a design concept. One person might type, “We need a concept for our sci-fi game level – maybe a futuristic cityscape,” and as the AI generates it, another can chime in, “Make the city floating in the clouds,” and so on. The image evolves as a live collaborative product of the conversation. This kind of interaction was much harder when using standalone prompt-based systems.
Of course, these possibilities also raise questions: Will this make creative work too dependent on AI’s suggestions? How do we balance the AI’s input with human imagination? These are open-ended questions, but the convergence of image generation and language models is undeniably expanding how we create and communicate visually. Next, let’s look at some concrete domains where these AI image generation tools are making waves right now, and how they might shape the near future.
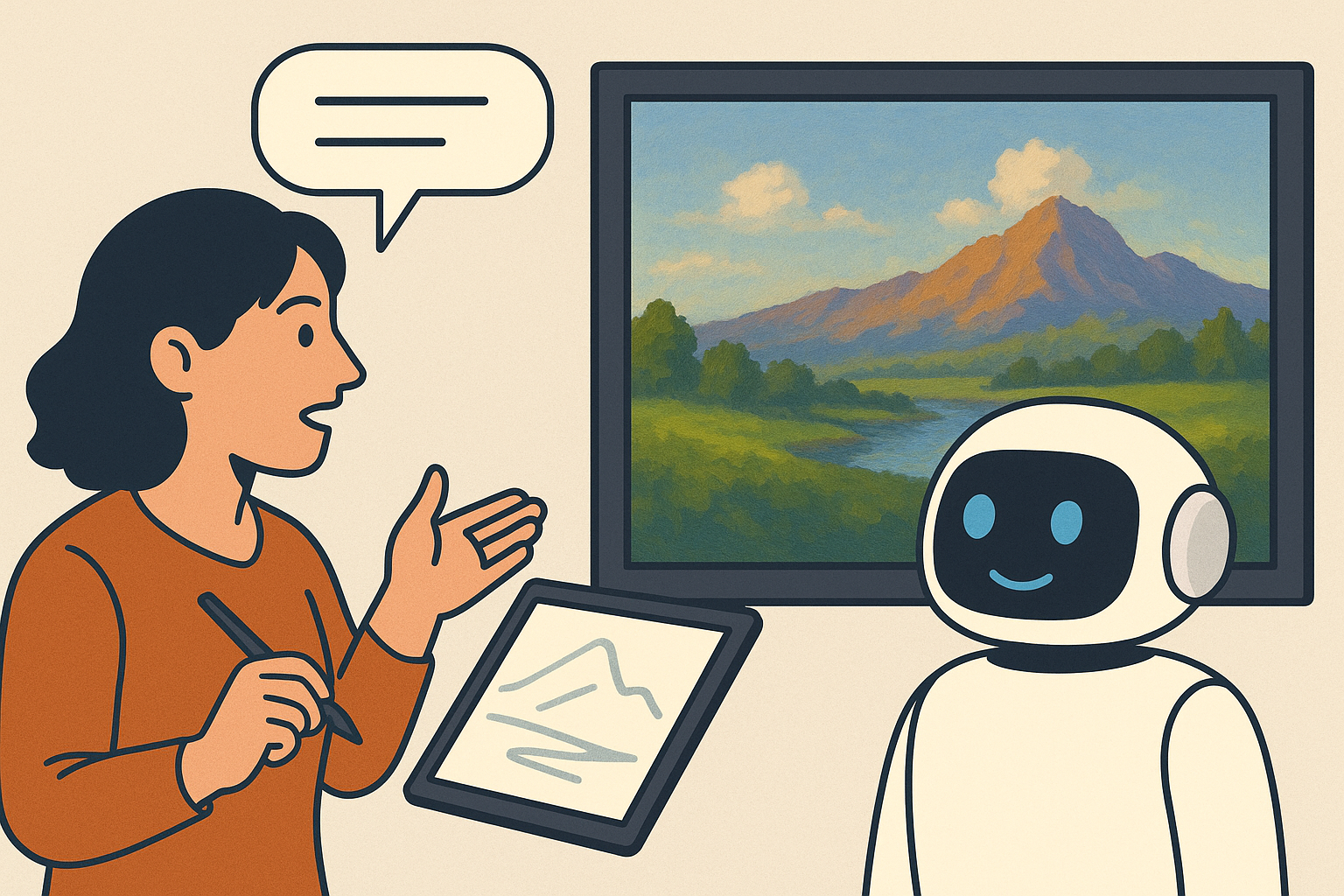
Real-World Applications in 2025
AI image generation is not just a neat toy – it’s being put to work across various industries and everyday scenarios. Let’s explore a few key areas (marketing, gaming, education, and accessibility) where these models are having an impact:
Marketing & Advertising
In marketing, visual content is king, and AI image generators are helping produce that content faster than ever. Agencies and brands are using tools like Stable Diffusion and DALL·E to generate campaign visuals, product concept images, and even entire ads. Need an image of a new sneaker design on a splashy background? Type it in, and you get multiple variations to choose from. This allows for rapid A/B testing of visuals: marketers can quickly get several ad mockups and see which one resonates best, before committing to a full photoshoot. Big companies have noticed this potential – for instance, Coca-Cola announced a partnership to explore using OpenAI’s generative models in their marketing content creation. And it’s not just prototypes; we’ve seen real campaigns use AI-generated art. One cosmetics brand used Midjourney to create a series of surreal product images for Instagram, catching audience attention with visuals that would be costly or impossible to set up physically.
AI also enables personalization in advertising. A travel agency could generate on-the-fly images of a destination with a customer’s name written in the sand or formed in clouds, tailoring the visual to each viewer. Previously, making 100 different personalized images would be impractical – now it’s feasible. Creative teams are incorporating these models into their workflow, often via tools integrated in software they already use (Adobe’s tools, Canva, etc., are adding AI image generation features). It’s important to note, however, that human oversight remains crucial. Marketers must ensure the AI isn’t inadvertently copying someone’s artwork or inserting odd details. There have been instances where a generated image had a distorted company logo or nonsensical text in the background – little tells that an AI made it. So, teams are learning to use AI as a powerful assistant, but with a careful review process, treating it as a brainstorming and prototyping aid. The result is a significantly accelerated creative cycle for advertising, where ideas move from concept to visualization with unprecedented speed.
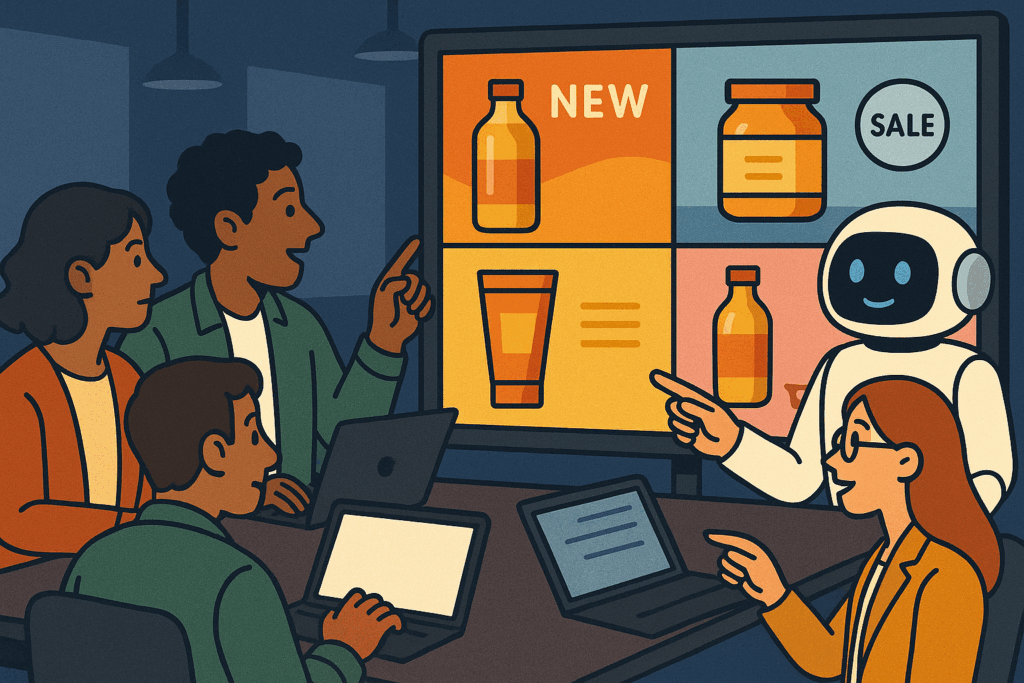
Gaming & Entertainment
The gaming industry has eagerly embraced AI-generated imagery, as it aligns perfectly with the need for vast amounts of art in game development. Concept artists at game studios use diffusion models to generate quick visuals of characters, creatures, and environments. These AI-generated concepts serve as a starting point, which artists can then refine and paint over. For example, if you need 50 ideas for alien creatures for a sci-fi game, an AI can produce a gallery of bizarre and creative creature designs overnight. The art team can pick the closest ones and refine the anatomy or style rather than drawing everything from scratch. This doesn’t replace the artists – rather, it turbocharges the early creative phase. Some indie game developers with small teams even use AI art directly for certain assets (though this can be controversial if not done carefully). We’ve seen indie projects generate their background illustrations or item icons with tools like Midjourney to save time and money.
Beyond development, imagine in-game AI generation. We’re not far from games where the player can type a description and the game generates a custom item or paints a mural on a wall in the game environment dynamically. There’s already a text adventure game prototype that creates scene images on the fly as you describe your actions. Autoregressive multimodal models might make this smoother, understanding the game context via text and producing matching visuals in real-time. This could lead to extremely personalized gaming experiences – the game’s art adapts to each player’s imagination.
In film and entertainment, similar patterns are emerging. Storyboard artists can generate storyboards from scripts using AI images, making it easier to visualize scenes before investing in expensive sets or CGI. There was a short film in 2023 that famously used AI-generated backgrounds for scenes, showcasing how a small film crew could achieve big-budget-looking sets by blending actors with AI art backgrounds. While that sparked debate about the future of human set designers and artists, it proved the concept that AI can contribute to the visual creation in movies. We can expect more experimentation here: maybe a director will use AI to quickly prototype costume designs or to visualize different color gradings for a scene. As the tools improve, the line between pre-production (planning visuals) and production might blur – AI could even generate some final assets under human artistic direction.
Education & Learning
Education is another area getting a boost from image generation models. Teachers and educators can use these tools to create custom illustrations and visuals for their lessons. Instead of searching for the perfect image in textbooks or online, a teacher can simply ask an AI to generate what they need. Picture a history teacher talking about ancient Rome – they could generate an image of a Roman marketplace to show the class, tailored to the specific setting they want to highlight. Or a biology teacher could conjure up a diagram of a cell with labels in a more engaging art style. This makes learning more visual and interactive, especially for subjects that benefit from diagrams or scene-setting. It’s like having an unlimited on-demand clipart and illustration library, where you just request what you need.
Students, too, can benefit. Think of a creative writing assignment: a student writes a short story and then uses an AI to illustrate their storybook. This can be incredibly motivating and fun – they see their ideas come to life visually. It can also aid understanding; for instance, if a student is struggling to imagine a concept, they could ask the AI to show it to them. Learning a new language? Generate images of vocabulary words to create flashcards or story scenes using those words. The multimodal models (like future GPT-based ones) could even explain the image they generated, reinforcing learning (e.g., the AI generates a picture of a volcanic eruption and also describes the science behind it in the lesson).
There are already platforms integrating AI art for education. Some educational apps let kids draw a doodle and have an AI “complete” it into a more finished artwork, combining creativity with surprise. Museums and online courses are generating bespoke images to illustrate content that might not have readily available visuals. That said, educators have to ensure accuracy – AIs might occasionally produce an incorrect detail (say, an anachronistic element in a historical scene or a mislabeled diagram). So fact-checking and guiding the AI with specific prompts is part of the process. Overall, AI image generation has the potential to make learning more engaging, personalized, and imaginative.
Accessibility & Inclusion
One of the most heartwarming applications of image generation AI is in improving accessibility and inclusion in creative expression. Traditionally, visual art creation has barriers – you might need fine motor skills, years of training, or expensive tools. AI is changing that by lowering the entry barrier. For people with physical disabilities, these tools can be liberating. There’s the example of a board game artist, Sean Aaberg, who suffered a stroke that limited his ability to draw by hand. He turned to AI (using Midjourney) to continue creating art for his projects, effectively reclaiming his artistic voice through a new medium. Similarly, individuals who cannot use their hands to draw or paint can use voice commands or assistive technologies in combination with AI to generate images. An artist with limited mobility could describe their vision out loud, and watch the AI produce a first draft. They can iterate verbally: “No, make the background darker” – and refine the piece. It’s a new form of assistive technology that empowers people to create visuals even if the traditional methods are not accessible to them.
AI image generators can also help those who lack formal art training or resources. Not everyone had the opportunity to learn painting techniques or afford software and hardware for digital art. Now, if you have access to the internet, you have access to a world-class “artistic assistant”. A person with ideas in their head can bring them to life without needing to master Photoshop or possess natural drawing talent. This is incredibly inclusive – more voices and visions can be expressed visually than before. We’re seeing disabled artists and hobbyists alike publish graphic novels, webcomics, or illustrated stories using AI for the visuals, something that might have been prohibitive before.
There’s also a flip side: AI can help consume visual information in accessible ways. For someone who is visually impaired, AI models (especially when paired with language models) can generate detailed descriptions of images or even tactile representations. For instance, an AI vision model could look at an image and generate a rich textual description that a screen reader can read aloud – effectively translating images to words. While that’s more about analysis than generation, it’s part of the same technological trend of bridging visual and textual content. In the future, the line might blur: maybe a blind user could ask a model to “draw” a scene and then describe it to them, creating a mental image via words.
In terms of inclusion, one challenge has been representation in generated images. Early AI models sometimes showed bias – e.g., prompting for a “doctor” might default to a male figure in many models, reflecting biases in training data. But as awareness grows, there’s an effort to make AI images more diverse and representative. Users can explicitly prompt for diversity (like “a group of engineers of different genders and ethnicities”) and get results that celebrate inclusivity. The hope is that these tools, if guided well, can help normalize seeing a wide range of people in various roles in media, by making it easy to create such images.
From marketing to gaming, education to accessibility, the current applications of AI image generation are impressive. They hint at a future where creating visual content is as commonplace as writing an email. But with great power comes great responsibility, and it’s important to weigh the opportunities against the risks and challenges as we move forward.